Environmental DNA (eDNA) analysis has emerged as a disruptive innovation in ecology. It involves the collection and analysis of DNA fragments found in the environment, such as water or soil, to study organisms and ecosystems. Effectively leveraging the massive datasets it produces requires embracing the principles and tools of big data. From high-performance computing to open data sharing, eDNA is driving rapid change in ecological research methodology.
The Genetic Data Deluge
A single eDNA sample can yield over 100 gigabytes of DNA sequencing data. Metabarcoding entire ecological communities generates tens of terabytes per study. This exponential growth mirrors other big data domains, presenting new opportunities and challenges.
Advanced sequencing technology has sent genomics data surging exponentially, doubling every 7 months. Likewise, eDNA data is skyrocketing as costs plummet and sampling expands. Workflow automation and parallel processing now enable industrial-scale sequencing of thousands of samples simultaneously[^3^].
But this firehose of complex biological data quickly overwhelms traditional analytical approaches. Successfully channeling these massive eDNA datasets to extract ecological insights hinges on adopting a big data mindset.
Leveraging Cloud Computing
Environmental DNA (eDNA) metabarcoding generates astronomical datasets with sequences numbering in the billions per study. Analyzing these vast genetic libraries to reveal ecological insights demands high-performance cloud infrastructure. Let’s examine how cloud computing is empowering a new era of accelerated eDNA discovery.
Related reading: The Benefits of Cloud Computing and Data Storage for Scientific Research
Surging Computing Needs
A single eDNA sample can produce over 100 gigabytes of DNA sequences. A large project encompassing thousands of samples spread across ecosystems easily generates terabytes of data requiring analysis. This rapidly exceeds storage and processing capacities of standard lab servers.
While sequencing costs have fallen dramatically, computational requirements keep rising. One estimate suggests including eDNA could increase computing needs for a typical ecology project by 100,000-fold! Desktop workstations and isolated research clusters are clearly inadequate.
Cloud Powers On-Demand Scalability
Cloud computing delivers the dynamic, large-scale infrastructure required for modern eDNA investigation. Cloud resources can be rapidly provisioned to tackle huge workloads and then released once projects complete. This elasticity enables aligning compute power with ever-changing analysis needs.
Services like Amazon EC2, Google Compute Engine, and Microsoft Azure provide access to virtual servers on demand. Parallel cloud processing accelarates eDNA workflows exponentially faster compared to standard sequential analysis.
Automated Bioinformatic Pipelines
Specialized platforms build on raw cloud infrastructure to offer pre-built pipelines for eDNA analysis. These automated workflows allow running a sequenced sample from raw reads through species identification and visualization with one click.
CyVerse provides the GigaScience eDNA pipeline to process next-generation sequencing data at massive scale using cloud parallelization. It coordinates quality control, sequence assembly, taxonomic classification, and visualizations.
MG-RAST offers automated ribosomal RNA analysis of eDNA samples by leveraging high-performance cloud resources. Machine learning aids species assignment for hundreds of samples simultaneously.
Shared Reference Databases
Centralized open databases hosted in the cloud empower collective eDNA investigation. Instead of each lab generating their own reference sequence library, shared community resources like GenBank provide universally available DNA barcodes.
Standardized resources prevent duplication of efforts and enable collaboration. Indexed cloud-based systems like Kraken 2 rapidly compare eDNA reads against genomic reference databases for quick taxonomic identification.
Democratized Access
Significant cloud adoption barriers for researchers include costs, technical skills, and data transfer needs. Initiatives like CyVerse and SciServer Launchpad lower hurdles by providing free cloud credits and access to preconfigured tools through intuitive interfaces.
CyVerse offers both command line and point-and-click gateways to cloud-based eDNA pipelines tailored for scientists without coding expertise. This democratization expands access and sharing of computational capabilities.
The Cloud Future
As eDNA datasets grow exponentially, cloud computing unlocks once unfathomable analysis capabilities. On-demand scalability, automation, and collaboration will accelerate eDNA insights to drive ecological discovery and conservation.
However, challenges remain around data movement, workflows, training, and costs. Thoughtful design of eDNA cyberinfrastructure will determine how quickly the field realizes the cloud’s disruptive potential. The future of eDNA resides in the cloud!
Related reading: The Benefits of Cloud Native Geospatial File Formats
Machine Learning in eDNA Analysis
Parsing endless DNA reads to identify source organisms is also benefiting from machine learning advances. Machine learning provides a scalable solution to handle relentless eDNA data flows.
Machine learning, particularly deep learning neural networks, is gaining traction in the processing and analysis of the vast sequence data generated by eDNA studies. This technology offers numerous benefits, including increased speed, accuracy, and automation. However, it’s essential to understand its potential challenges and limitations to ensure successful implementation in professional settings.
In species classification, convolutional neural networks (CNNs) have demonstrated their ability to quickly classify short DNA reads into taxonomic groups. For example, a 2021 study found that a CNN could accurately classify vertebrate eDNA samples to species with 84% accuracy, compared to 71% for traditional methods. Datasets with millions of eDNA sequences can be automatically classified through AI-powered tools like Metaxa and GENEIOUS Prime. These machine learning algorithms greatly accelerate the complex process of mapping reads to known taxa.
Additionally, in metagenomic analysis, a long short-term memory (LSTM) neural network called MetaML has been successful in classifying metagenomic microbe samples into taxa with 98% accuracy. This outperforms other classifiers and aids in annotating extensive microbiome datasets. Furthermore, generative neural networks have been utilized in designing novel PCR primers for eDNA analysis of mammals and fish, learning primer design patterns to output optimized candidates.
Machine learning offers several advantages for eDNA analysis. Its speed allows for the classification of sequence reads over 100 times faster than alignment-based methods, making it essential for handling large datasets. Deep learning algorithms can also improve classification accuracy by identifying complex patterns in sequence data that traditional algorithms may miss. Once trained, ML models can continuously analyze new data without human intervention, enabling automation. Moreover, these models can classify unseen species based on foundational patterns learned from training data.
Despite these benefits, machine learning also presents some challenges. The reasoning behind ML model predictions can be difficult to interpret, in contrast to transparent rules-based algorithms. Furthermore, models heavily rely on the quality of their training data, necessitating solid curated reference sequence datasets. Training complex ML models can also be computationally expensive, requiring significant cloud computing resources and time. Finally, models may overfit to idiosyncrasies in training data rather than generalizable patterns.
The Importance of Data Sharing
Shared Data Resources
Realizing eDNA’s potential relies on community data sharing, powered by standardized metadata, data formats, and public repositories.
Genetic reference databases like GenBank contain millions of DNA barcode sequences available for global use. Unified metadata standards like MIxS and Darwin Core facilitate open data discovery and integration.
Global Omics Observatory Network
Initiatives like Global Omics Observatory Network provide cloud-based portals to search across vast, distributed eDNA resources. Democratizing access allows networked collective intelligence to mine rich ecological insights.
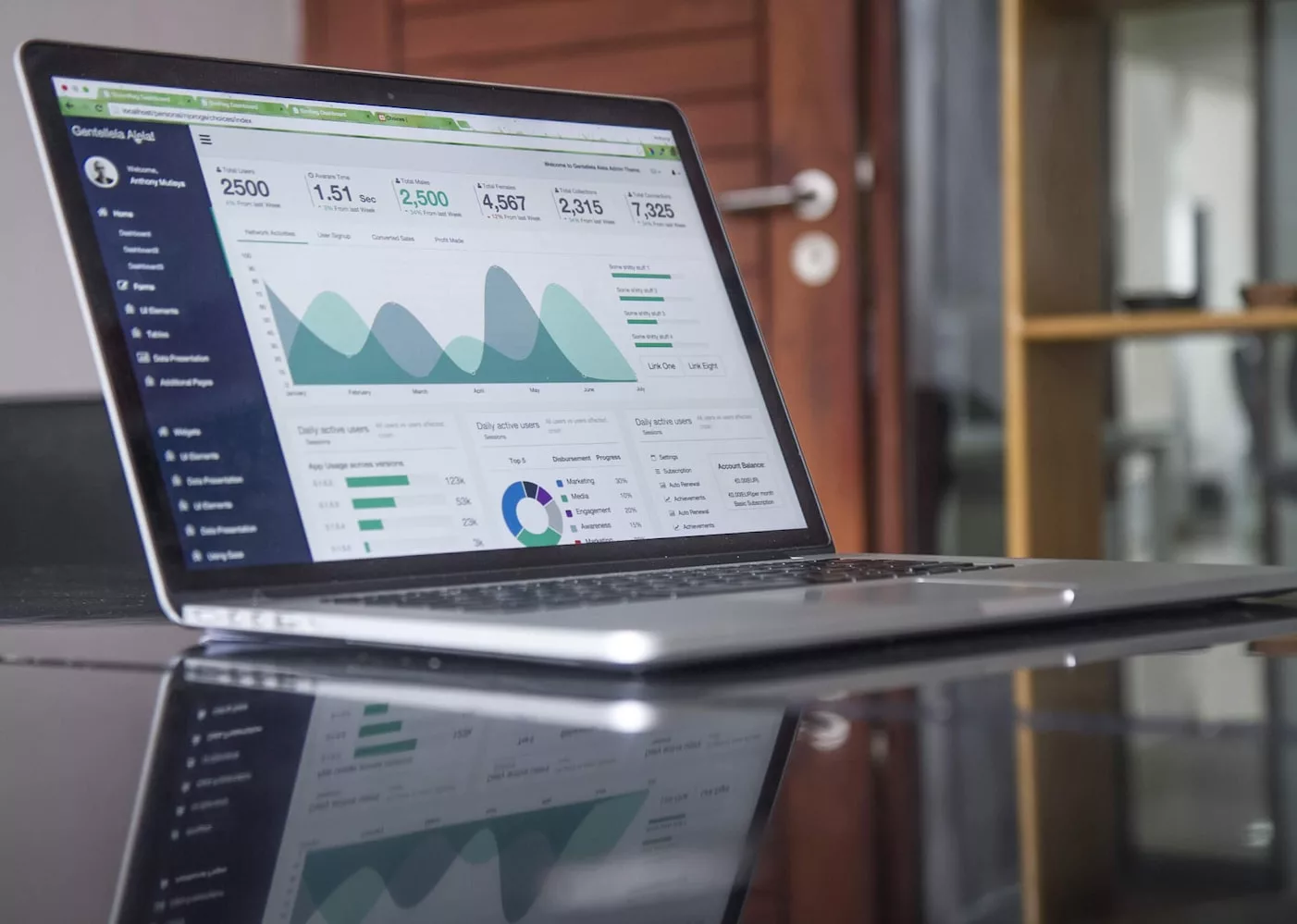
Synthesizing Diverse Environmental Data
Maximizing eDNA’s potential also requires synthesizing it with expansive data streams from sensor networks, earth observation, climatology, and more.
Integrating layered environmental data provides unparalleled spatio-temporal insights into ecological change. This necessitates developing advanced cyberinfrastructure to connect disparate data silos into knowledge-generating ecosystems.
As high-throughput eDNA analysis rapidly matures, it serves as a microcosm of the wider big data revolution transforming ecological research methodology.
Read more: Assessing Ecosystem Health with Environmental Analytics: A Step-by-Step Guide
Careers at the Intersection of Ecology and Big Data
This big data transformation is also creating new opportunities for data scientists, engineers, and computational experts in the ecology domain.
As the datasets grow in scale and complexity, cross-domain collaboration is increasingly essential. Experts in data engineering, machine learning, visualization, and software development are needed to propel eDNA capabilities forward.
The future of ecology resides at the intersection of biological expertise, environmental science, and big data capabilities. Exciting possibilities await this fusion!
Conclusion
The integration of eDNA analysis and big data is reshaping the field of ecology, offering unprecedented opportunities for insight and discovery. By embracing cloud computing, machine learning, and data sharing, researchers can harness the full potential of eDNA and revolutionize our understanding of the natural world.
Next Steps
Round Table Environmental Informatics (RTEI) is a consulting firm that helps our clients to leverage digital technologies for environmental analytics. We offer free consultations to discuss how we at RTEI can help you.